CVPR2023に論文が採択 Paper was accepted at CVPR 2023
2023.06.01
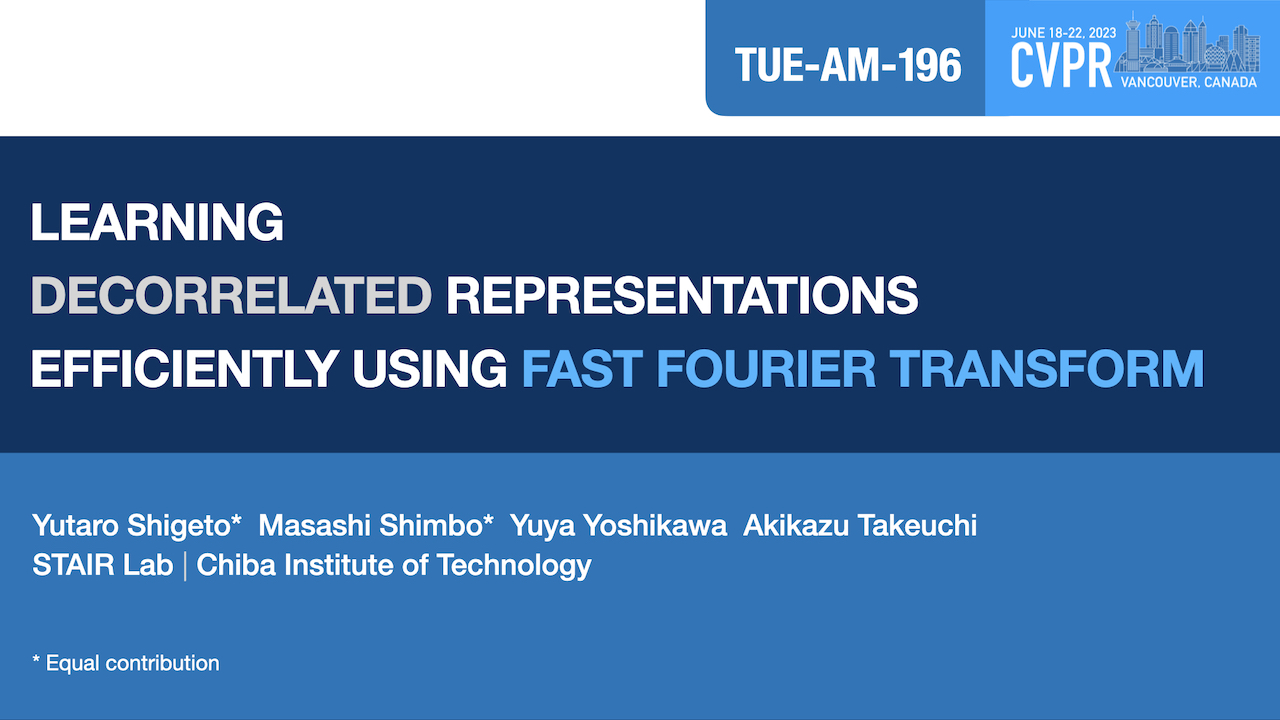
2023.06.01
コンピュータビジョンのトップカンファレンスであるCVPR 2023において下記の論文が採択されました。
【タイトル】
Learning Decorrelated Representations Efficiently Using Fast Fourier Transform (CVPR 2023)
【著者】
Yutaro Shigeto*, Masashi Shimbo*, Yuya Yoshikawa, Akikazu Takeuchi
* Equal contribution.
【概要】
Barlow Twins and VICReg are self-supervised representation learning models that use regularizers to decorrelate features. Although these models are as effective as conventional representation learning models, their training can be computationally demanding if the dimension d of the projected embeddings is high. As the regularizers are defined in terms of individual elements of a cross-correlation or covariance matrix, computing the loss for n samples takes O(n d2) time. In this paper, we propose a relaxed decorrelating regularizer that can be computed in O(n d log d) time by Fast Fourier Transform. We also propose an inexpensive technique to mitigate undesirable local minima that develop with the relaxation. The proposed regularizer exhibits accuracy comparable to that of existing regularizers in downstream tasks, whereas their training requires less memory and is faster for large d. The source code is available.