ZOZO研究所との共同研究論文がIJCAI2025に採択 A collaborative research paper with ZOZO Research accepted at IJCAI 2025
2025.05.26
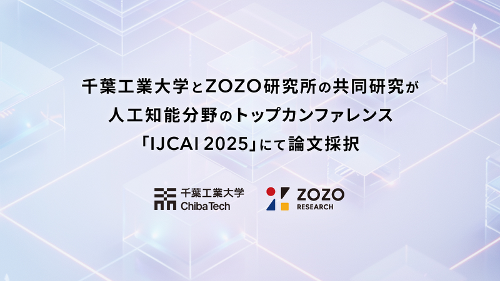
2025.05.26
弊センターの吉川と、メルボルン大学 木村 正成氏、ZOZO研究所の清水 良太郎氏、斎藤 侑輝氏とで行った共同研究により執筆した論文、”Explaining Black-box Model Predictions via Two-level Nested Feature Attributions with Consistency Property”がIJCAI2025に採択されました。
The paper “Explaining Black-box Model Predictions via Two-level Nested Feature Attributions with Consistency Property,” authored through collaborative research by Yoshikawa from our center, Masanari Kimura from the University of Melbourne, and Ryotaro Shimizu and Yuki Saito from ZOZO Research, has been accepted at IJCAI 2025.
AIが予測を行った根拠を説明することの重要性が高まっていますが、複数の要素をまとめて解析するマルチインスタンス学習(MIL)では、説明が特に難しい課題となっています。従来の手法では、「どの要素が重要か(HiFAs)」と「その要素内でどの特徴が重要か(LoFAs)」を個別に推定していたため、計算負担が大きくなり、説明内容に不整合が生じることが問題でした。本研究では、この問題を解決するため、新しい手法「C2FA(Consistent Two-level Feature Attribution)」を提案しています。C2FAは、HiFAsとLoFAsを同時に推定することで両者の一貫性を保ち、交互方向乗数法(ADMM)を使って効率よく計算します。実験の結果、従来法よりも少ない計算回数で、高精度かつ視覚的にも一貫した説明を提供できることが確認できました。
While the importance of explaining AI model predictions continues to grow, it remains particularly challenging in multi-instance learning (MIL), which involves analyzing groups of multiple instances simultaneously. Existing methods separately estimate “High-level Feature Attributions (HiFAs),” determining which instances are important, and “Low-level Feature Attributions (LoFAs),” identifying crucial features within these instances. However, these methods are computationally intensive and lead to inconsistencies between explanations.
In this research, we propose a novel approach called “C2FA (Consistent Two-level Feature Attribution)” to address these issues. C2FA simultaneously estimates HiFAs and LoFAs, ensuring consistency between both levels, and efficiently computes attributions using the Alternating Direction Method of Multipliers (ADMM). Experiments confirmed that C2FA provides more accurate and visually consistent explanations than conventional methods, achieving these results with significantly fewer computations.
IJCAI2025に採択された論文のExtended versionが、こちらからご覧いただけます。
また、プレスリリースの内容はこちらからご覧いただけます。
The extended version of the accepted IJCAI 2025 paper is available.